[dropcap style=”font-size:100px; color:#992211;”]G[/dropcap]iven that high frequency trading means the markets are already controlled by algorithms, adding a collapse predictor sequence seems somewhat tautological.
Isn’t it time we started basing our economies on a German octopus?
The learning mechanism of neurones has inspired researchers at the University of Valladolid (Spain) to create algorithms that can predict whether a bank will go bust. The model was correct for 96% of the banks that went bust in the USA in 2013 after analysing their financial indicators from the previous decade, marked by the economic crisis. The most vulnerable were those which had accumulated loans from the construction sector and grown rapidly without sufficient provisions.
Since the economic crisis started in 2008 more than 300 banks have gone bust in the US, a country which has an extensive database of its 7,000 financial entities and where bankruptcies are published daily. This information, provided by the Federal Deposit Insurance Corporation, has helped to validate the model which two economists from the University of Valladolid (Spain) have developed to calculate the probability of banks going bust.
The researchers used financial indicators or ratios from North-American entities for the period between 2002 and 2012. From this data and operating in a different time period, the model managed to deduce how many would go bankrupt between May 2012 and December 2013. According to the study published in the journal ‘Expert Systems with Applications‘, the results were correct 96% of the time.
“There are many analyses which can predict bankruptcy one year in advance, which is too short a timeframe to take preventative action,” as one of the authors, Iván Pastor, tells SINC. Pastor highlights the predictive capacity of their short, medium and long-term algorithms, which relate respectively to one, two and three years.
The researcher points out that they created the model using neuronal networks, a set of algorithms which function by imitating the behaviour of the human nervous system and which are very useful in detecting patterns. These are what predict the possibility of bankruptcy.
“This methodology also generates a two-dimensional map which will help bank authorities and regulators to visualise the whole financial system and identify problematic entities in the very short-term, as well as solvent entities which could present problems in the long-term,” says Pastor.
Thanks to these analyses the different routes which could lead a financial entity to bankruptcy could also be observed. By applying the model it is clear that those which presented a greater risk were those with a high concentration of construction-related loans, those which grew very rapidly, did not have a proper market capitalisation and had low levels of provisions.
Potential to predict for other dates and countries
“The results that we obtained for December 2013 demonstrated that the US financial system had improved compared to the darkest moments of the economic crisis, but there were still entities at high risk of going bankrupt, although they were generally minor entities,” adds Pastor, who recognises that the model has not yet been updated with 2015 data, “although, given enough time, it could be done”.
The expert confirms that this methodology could be applied to learn the bankruptcy potential of financial entities in other countries, with the necessary readjustments and modifications to each nation’s characteristics.
“For example, some financial ratios employed in the US are not available in Spain, given that less information is public in our country,” adds Pastor, although he comments on another study currently in progress: “We are waiting for a study to be published on Spanish savings banks, where we analyse how many of them ended up bankrupt or bailed out, as well as identify the factors differentiating healthy and failed entities”.
Source: FECYT – Spanish Foundation for Science and Technology
Image: reynermedia
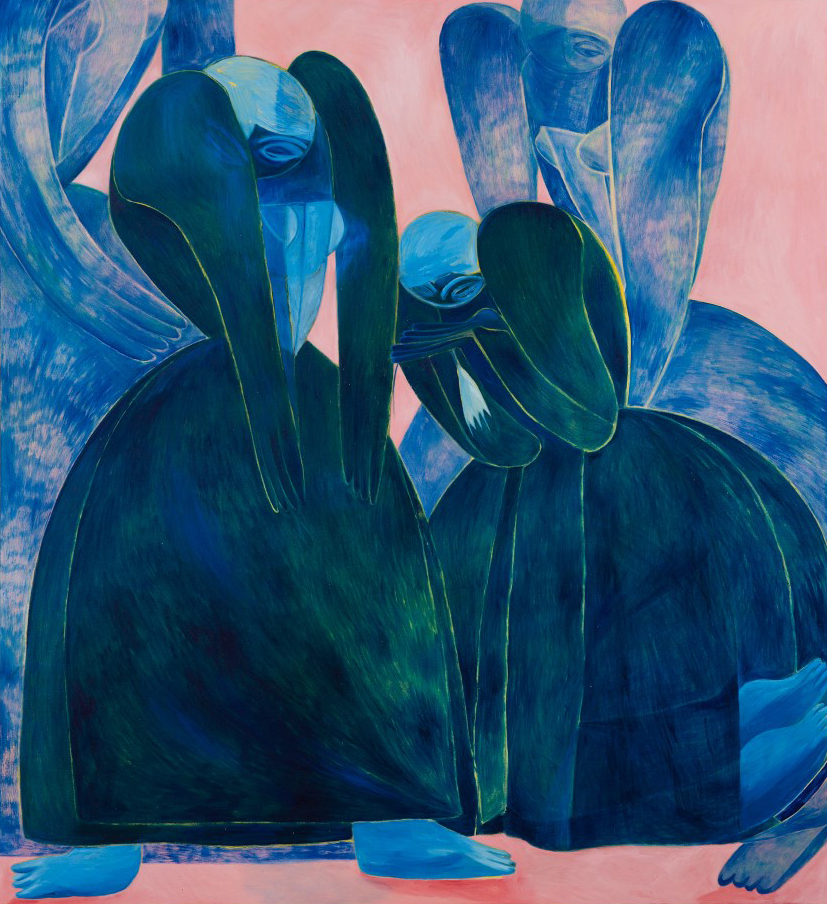
Some of the news that we find inspiring, diverting, wrong or so very right.