[dropcap style=”font-size:100px;color:#992211;]R[/dropcap]ichard Wollheim, some-time Grote Professor of Mind and Logic at University College London, regards our looking at pictures as a special kind of undertaking.
In our experience of pictures we seem to see two things at once.
The single complex experience, according to Wollheim, has two folds. The first fold is the configurational: we see the flat patterned surface of the picture. The second fold is the recognitional: we see in that flat patterned surface, the three-dimensional world comprising objects, scenes and events.
According to Wollheim, the complex experience is perceptual in both its folds. We see landscapes, for instance, in our seeing the surfaces of pictures.
Other contemporary philosophers hold a slightly different view. They argue that seeing the surface is somehow an occasion for our imagining the landscape on the basis of the pattern.
Seeing the pattern is a straightforward perceptual ability. The further experience, based on that perception is an imaginative construction of the scene ‘seen’. It is as if a mental image of the scene converges with the perception of the surface. It is this convergence that comprises pictures and pictorial seeing.
Recent findings in the research publication, Proceedings of the National Academy of Sciences, published on May 29, 2018, confirms that there are gifted professional face recognisers who match images of faces across pairs consistently better than other humans. The current state of Artificial Intelligence applied to facial recognition suggests that professional humans do about as well as computer generated algorithm based face recognition.
Surprisingly, the authors conclude, the best results were obtained when professional face recognisers teamed up with AI systems.
One of the researchers, NIST electronic engineer P. Jonathon Phillips, said,
If combining decisions from two sources increases accuracy, then this method demonstrates the existence of different strategies. But it does not explain how the strategies are different.
It is at least compatible with the view that when we see faces in pictures we do so imaginatively. Like seeing faces in the clouds, the experience is of a three-dimensional head and not like seeing an internal picture – a flat thing.
When I see a face in the clouds an imagine of it that it is the head of an old woman I imagine her turning her rotating her head or moving it up or down. That is different from seeing it as flat and imagining the flat picture rotating.
If this is so, there is hope that we can think in terms of mental imagery as a bringing into mind the very objects and scenes we imagine; not pictures of them. We have, as it were, mental landscapes, inhabited by persons real or unreal, but whose habitation of the imaginary space is as it would be in a perceived world: three-dimensional.
One explanation for the enhanced results of human expert and AI partnership is that each brings a different means of solving the task.
If, as many philosophers believe, perception is to be explained in causal terms, it would explain why AI works purely on mechanical perceptual data derived from the two-dimensional surface of the pictures.
On the other hand, human experts can build into their method an imaginary picture of the three-dimensional head.
That is to say that the algorithms work on flat patterns and the humans work on something like three-dimensional models seen into the flat surfaces: pictures. And so imagination is recruited by hiumans in their reception of pictures.
________________________________________________________________________________
NIST study shows face recognition experts perform better with AI as partner
Multidisciplinary study provides scientific underpinnings for accuracy of forensic facial identification

CREDIT: J. STOUGHTON/NIST
Experts at recognizing faces often play a crucial role in criminal cases. A photo from a security camera can mean prison or freedom for a defendant–and testimony from highly trained forensic face examiners informs the jury whether that image actually depicts the accused. Just how good are facial recognition experts? Would artificial intelligence help?
A study appearing today in the Proceedings of the National Academy of Sciences has brought answers. In work that combines forensic science with psychology and computer vision research, a team of scientists from the National Institute of Standards and Technology (NIST) and three universities has tested the accuracy of professional face identifiers, providing at least one revelation that surprised even the researchers: Trained human beings perform best with a computer as a partner, not another person.
“This is the first study to measure face identification accuracy for professional forensic facial examiners, working under circumstances that apply in real-world casework,” said NIST electronic engineer P. Jonathon Phillips. “Our deeper goal was to find better ways to increase the accuracy of forensic facial comparisons.”
The team’s effort began in response to a 2009 report by the National Research Council, “Strengthening Forensic Science in the United States: A Path Forward”, which underscored the need to measure the accuracy of forensic examiner decisions.
The NIST study is the most comprehensive examination to date of face identification performance across a large, varied group of people. The study also examines the best technology as well, comparing the accuracy of state-of-the-art face recognition algorithms to human experts.
Their result from this classic confrontation of human versus machine? Neither gets the best results alone. Maximum accuracy was achieved with a collaboration between the two.
“Societies rely on the expertise and training of professional forensic facial examiners, because their judgments are thought to be best,” said co-author Alice O’Toole, a professor of cognitive science at the University of Texas at Dallas. “However, we learned that to get the most highly accurate face identification, we should combine the strengths of humans and machines.”
The results arrive at a timely moment in the development of facial recognition technology, which has been advancing for decades, but has only very recently attained competence approaching that of top-performing humans.
“If we had done this study three years ago, the best computer algorithm’s performance would have been comparable to an average untrained student,” Phillips said. “Nowadays, state-of-the-art algorithms perform as well as a highly trained professional.”
The study itself involved a total of 184 participants, a large number for an experiment of this type. Eighty-seven were trained professional facial examiners, while 13 were “super recognizers,” a term implying exceptional natural ability. The remaining 84–the control groups–included 53 fingerprint examiners and 31 undergraduate students, none of whom had training in facial comparisons.
For the test, the participants received 20 pairs of face images and rated the likelihood of each pair being the same person on a seven-point scale. The research team intentionally selected extremely challenging pairs, using images taken with limited control of illumination, expression and appearance. They then tested four of the latest computerized facial recognition algorithms, all developed between 2015 and 2017, using the same image pairs.
Three of the algorithms were developed by Rama Chellappa, a professor of electrical and computer engineering at the University of Maryland, and his team, who contributed to the study. The algorithms were trained to work in general face recognition situations and were applied without modification to the image sets.
One of the findings was unsurprising but significant to the justice system: The trained professionals did significantly better than the untrained control groups. This result established the superior ability of the trained examiners, thus providing for the first time a scientific basis for their testimony in court.
The algorithms also acquitted themselves well, as might be expected from the steady improvement in algorithm performance over the past few years.
What raised the team’s collective eyebrows regarded the performance of multiple examiners. The team discovered that combining the opinions of multiple forensic face examiners did not bring the most accurate results.
“Our data show that the best results come from a single facial examiner working with a single top-performing algorithm,” Phillips said. “While combining two human examiners does improve accuracy, it’s not as good as combining one examiner and the best algorithm.”
Combining examiners and AI is not currently used in real-world forensic casework. While this study did not explicitly test this fusion of examiners and AI in such an operational forensic environment, results provide an roadmap for improving the accuracy of face identification in future systems.
While the three-year project has revealed that humans and algorithms use different approaches to compare faces, it poses a tantalizing question to other scientists: Just what is the underlying distinction between the human and the algorithmic approach?
“If combining decisions from two sources increases accuracy, then this method demonstrates the existence of different strategies,” Phillips said. “But it does not explain how the strategies are different.”
###
The research team also included psychologist David White from Australia’s University of New South Wales.
________________________________________________________________________________
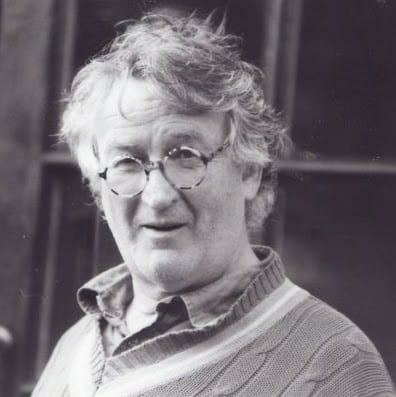
Ed studied painting at the Slade School of Fine Art and later wrote his PhD in Philosophy at UCL. He has written extensively on the visual arts and is presently writing a book on everyday aesthetics. He is an elected member of the International Association of Art Critics (AICA). He taught at University of Westminster and at University of Kent and he continues to make art.